3 ways insurers are using automated validations to gain a trustworthy view of their data
3 ways insurers are using automated validations to gain a trustworthy view of their data
3 ways insurers are using automated validations to gain a trustworthy view of their data
Validation rules allow you to create flexible queries, such as checking that all the values in a column have the same currency code, or warning when claims exceed a specified amount.
This is a simple yet powerful way to rapidly assess the quality of your large-scale datasets and the accuracy of your transformations. In fact, validations increased data confidence to such a degree for Aegon Blue Square Re that it allowed insights from their data to be used to set critical pricing assumptions.

Quantemplate has given us the data confidence needed to take action with the insights we discover.
Danny Verboom
Head of Operations — Aegon Blue Square Re
Here are three ways 3 ways insurers are using validations to build data confidence.
1. Get an overall view of data quality
The Validation Report provides a summary of the outcome of your validation rules, allowing you to assess data quality at a quick glance, rather than having to jump into the data to perform a series of manual sanity checks.
The Validation Report helps you set and meet benchmarks for data quality, both within your organisation and with your data suppliers.
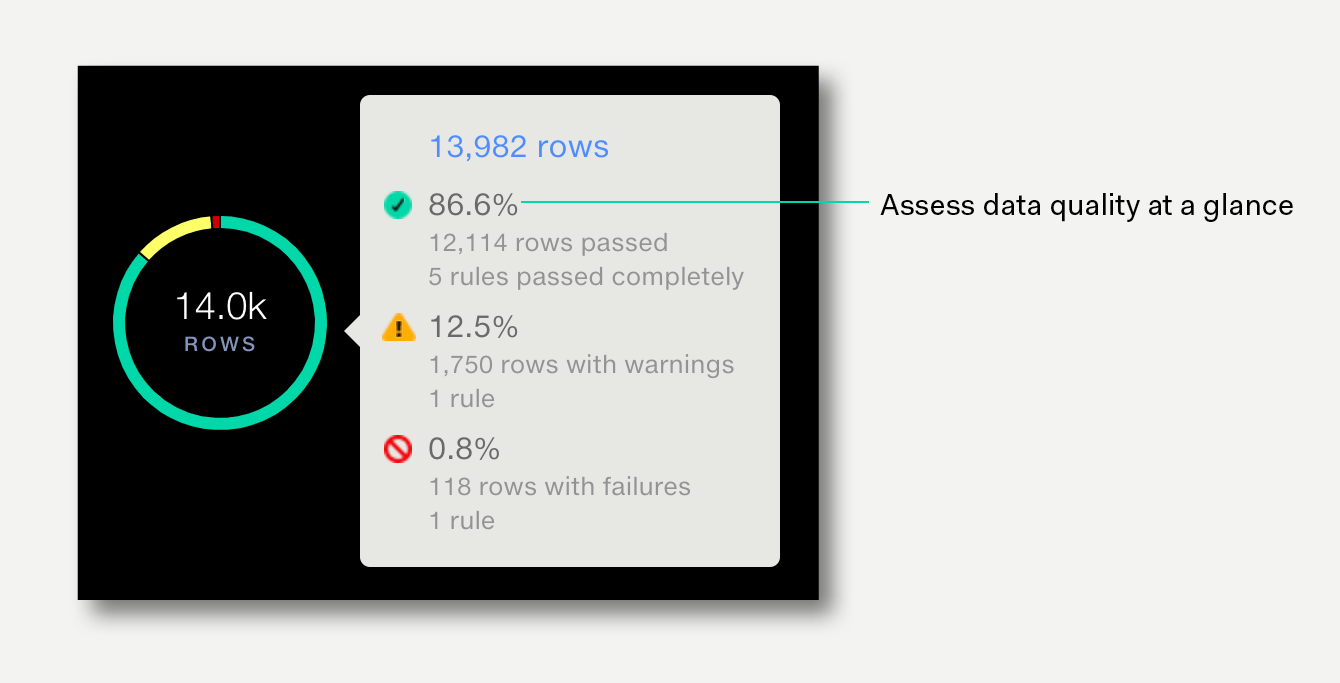
2. Instantly identify problem rows
We recently extended the validation suite capability to include the much requested drill down feature. Now you can instantly drill down to rows and columns that have failed data quality rules and quickly get to the source of the problem.
3. Grow confidence, even with complex transformations
With vast volumes of data passing through a set of automated processes, how can you be sure the output is correct? For example, an Aggregate operation enables the merging of rows, allowing you to roll-up data to point-in-time by reporting period, or to calculate totals and counts across a data set.
However, inconsistencies in your source data can result in merge failures which are almost impossible to detect when working with large datasets – and potentially very costly. That’s where validations help, automatically confirming your configuration, providing a report of rows which failed to merge as expected.
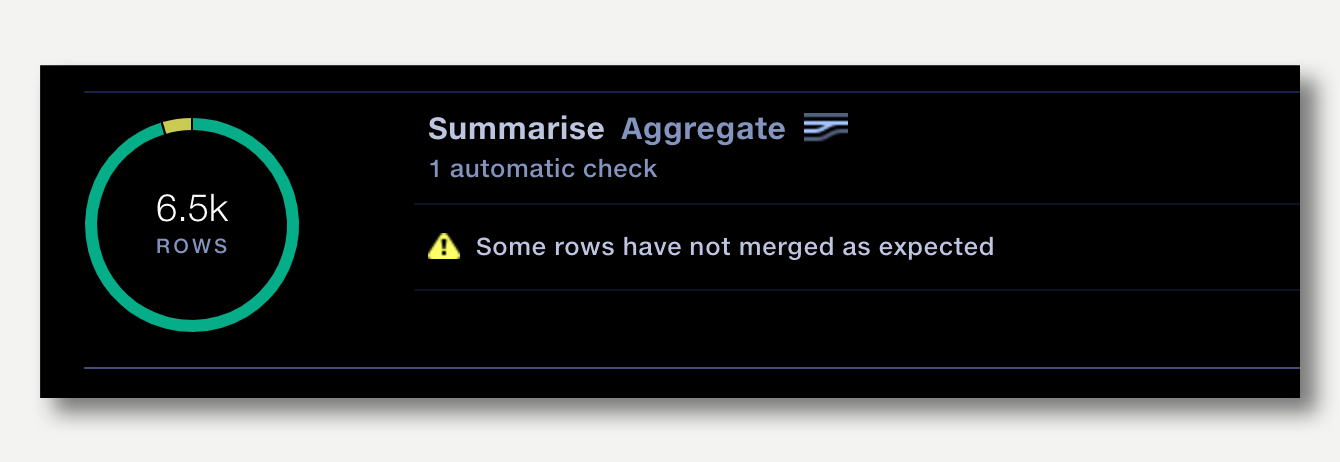
Get in touch to learn how your organisation could benefit from using validations when transforming data
Find out more about this story here:
Read more >